Foundations of Autonomy: Defining and Measuring Agency in AI Systems
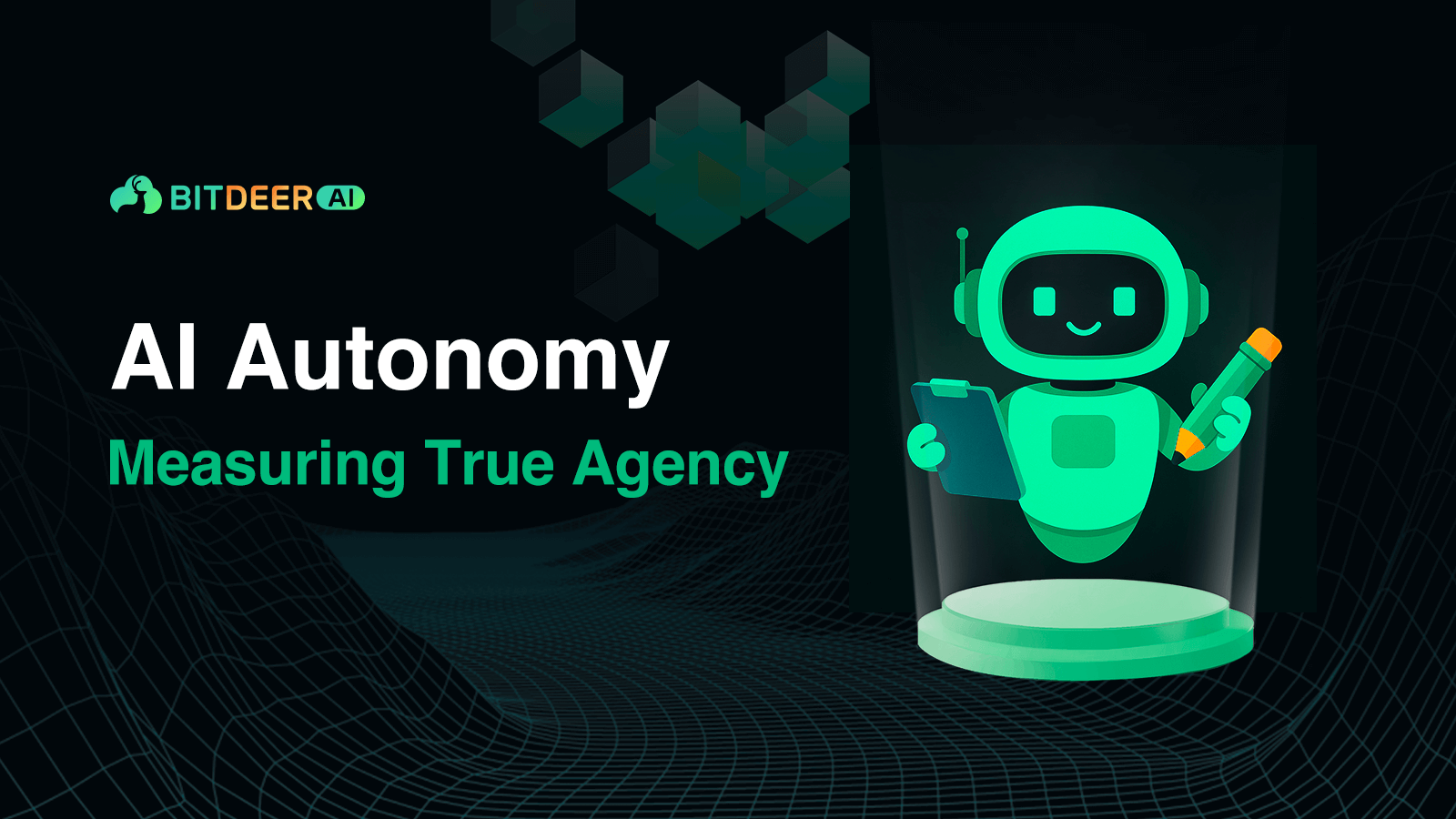
As artificial intelligence becomes increasingly woven into the fabric of our daily lives, the discussion around AI autonomy, often described as “agency”, has taken on new urgency. Agency refers to the ability of an AI system to act independently, make decisions, and execute plans without constant human oversight. While we casually refer to some systems as “autonomous,” digging deeper reveals a complex web of technical, philosophical, and ethical questions. We’ve published an article about key technical components of achieving agentic AI. But what does it really mean for an AI to have agency? How can we measure this autonomy, and what are its broader implications for society and the business world? This article seeks to unpack the foundations of AI agency and explore methods for defining and quantifying it, along with demonstrating real-world business use cases.
The Concept of Agency in AI
Agency is a well-established concept in philosophy and cognitive science, traditionally associated with beings capable of independent action and intentionality. When we bring this concept into the realm of AI, we confront a challenging question: can a machine truly be autonomous, or is it merely following a sophisticated set of instructions? At the heart of this debate is the tension between human-like understanding and mechanistic processing. AI systems today, from self-driving cars to robotic assistants, exhibit degrees of autonomy but often lack the self-aware intentionality that defines human agency.
Even a limited form of agency can dramatically alter how these systems interact with the world. Consider an autonomous drone that adapts its flight plan based on real-time obstacles, or a chatbot that seamlessly adjusts its responses in a customer service context. Defining this agency, whether as a spectrum or a set of discrete categories, is crucial for both the development and regulation of AI.
How Do We Measure Agency?
Measuring the autonomy of an AI system is no small feat. Researchers have proposed various frameworks to quantify agency, each attempting to capture different aspects of what it means for a system to act on its own:
- Decision-Making Independence: One metric involves evaluating how much of a system’s decisions are made without human intervention. In controlled environments, an AI might navigate dynamically changing scenarios while minimizing manual override.
- Goal-Directed Behavior: This assesses how effectively an AI can set and pursue its own objectives. It might involve testing the system’s ability to adjust its strategy when unforeseen circumstances arise.
- Adaptation and Learning: Monitoring an AI’s capacity to learn from experience and adjust its behavior over time is central to accurate outputs. Here, “lifelong learning” models come into play as they continuously refine their strategies based on new data.
- Transparency and Explainability: Finally, some measurement models emphasize how clearly an AI can explain its decision-making process. In scenarios where accountability matters, systems that articulate their reasoning provide increased trust and reliability.
Integrating these metrics offers a more comprehensive view of an AI system’s autonomy, guiding its development and informing stakeholders about its real-world capabilities.
Business Use Cases
Understanding and measuring AI agency isn’t just a theoretical exercise, it has significant practical implications for businesses:
- Autonomous Supply Chain Management: Companies can deploy AI systems that independently manage logistics, optimize delivery routes in real time, and respond adaptively to disruptions. These systems measure their own decision-making against established benchmarks, ensuring efficiency and reducing operational costs.
- Intelligent Customer Engagement: In customer service, agentic AI can take the lead on resolving complex queries with minimal human intervention. Such systems adaptively learn from customer interactions, tailoring responses and even escalating issues when necessary while providing a transparent record of the decision process.
- Autonomous Financial Trading: Investment firms use AI to analyze market data in real time, make buy-sell decisions, and adapt strategies based on evolving economic conditions. By quantifying aspects of agency like decision independence and goal-directed behavior, these systems can be trusted to operate with minimal oversight while adhering to strict regulatory guidelines.
The Philosophical and Ethical Dimensions
The challenge of defining agency in AI goes beyond technical metrics, it touches on deep philosophical questions about the nature of mind, intention, and ethical responsibility. If an AI can truly act autonomously, to what extent should it be held accountable for its actions? Could we or should we ever regard an AI as having “moral agency”? These questions have significant implications for law, policy, and societal trust, especially when businesses rely on autonomous systems for critical operations.
Toward a Standard Framework
One promising direction is the development of standardized benchmarks and evaluation protocols that can be applied across different types of autonomous systems. Such frameworks could help regulators, developers, and business leaders understand the capabilities and limitations of agentic AI. Standards might incorporate tests for adaptability, decision-making quality, and transparency, providing a clear picture of where an AI stands on the autonomy spectrum. Establishing such benchmarks is not only a technical challenge but also a collaborative effort involving diverse fields to ensure that the measures are both rigorous and fair.
The quest to define and measure agency in AI systems is one of the most exciting and challenging frontiers in modern artificial intelligence. It forces us to confront what it means to be autonomous, to act independently, and ultimately to make decisions that have a real impact on the world. As researchers develop more sophisticated tools and frameworks to evaluate AI autonomy, we inch closer to understanding the true potential and the limits of artificial agents. This knowledge is invaluable for businesses seeking to harness autonomous systems for competitive advantage while ensuring that ethical standards and transparency remain at the forefront of technological innovation. Embracing this journey means not only advancing the technical frontier but also aligning these breakthroughs with broader societal values.