The Application of RAG in Business Knowledge Management and Decision-Making
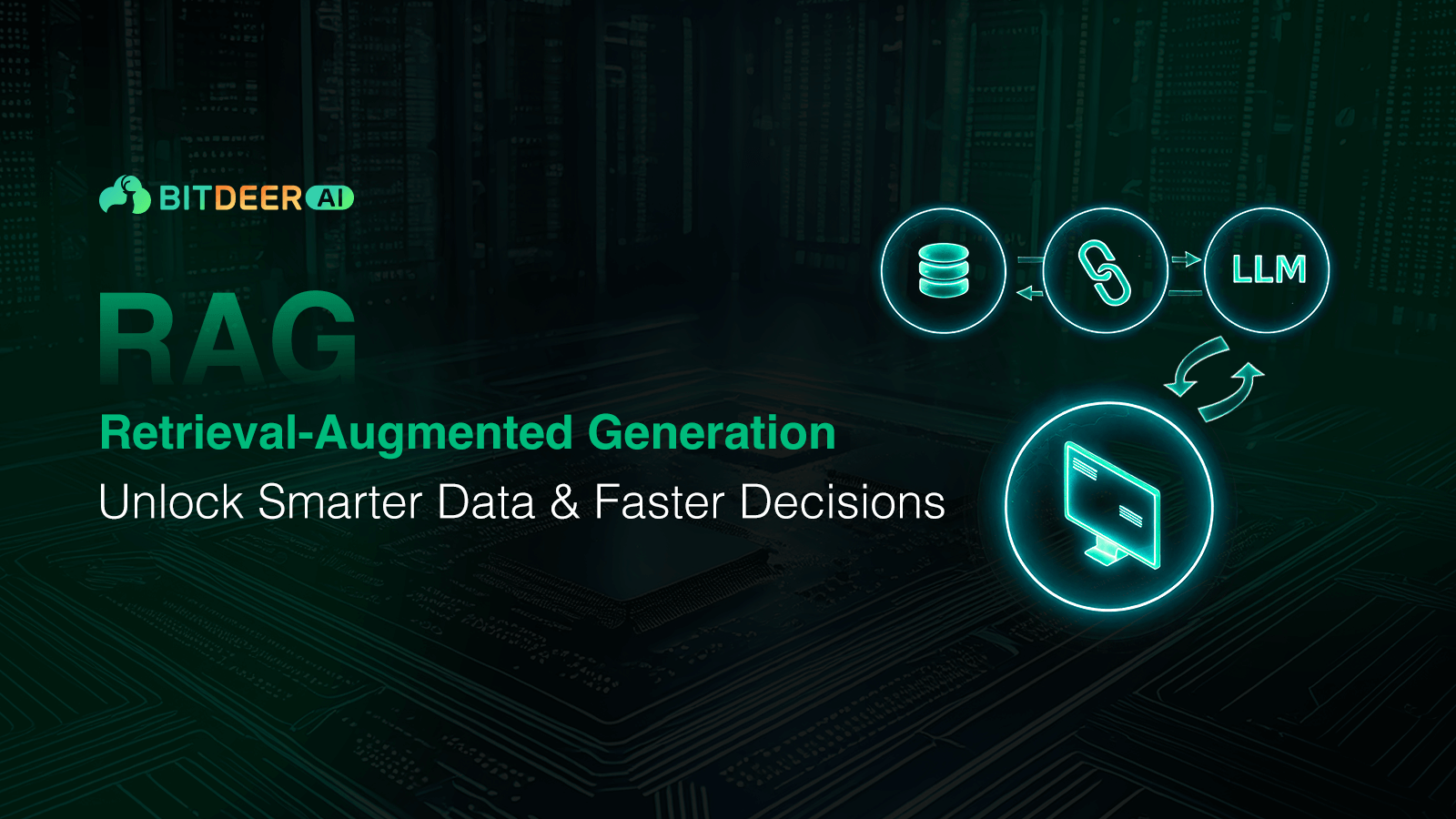
In an era of rapid information development and increasingly intense competition, a company's success may hinge on how quickly it responds to external changes—or even on how efficiently users perceive its solutions. Ultimately, the ability to extract the most relevant and optimal information from vast amounts of data, faster and more accurately, is crucial.
Businesses generate vast amounts of data daily, from emails and internal documents to customer interactions and market research. However, fragmented systems create information silos, making data retrieval, analysis, and application inefficient. Traditional AI models rely on static knowledge, lacking real-time, context-aware insights, leading to delays and poor decision-making.
Retrieval-Augmented Generation (RAG) offers a breakthrough in enterprise knowledge management by combining real-time information retrieval with generative AI. It enables businesses to quickly extract key insights from vast datasets, especially unstructured text, and apply them to strategic decisions. RAG helps companies respond faster to market changes, optimize operations, and build agile data management systems.
What is RAG?
RAG is an advanced AI framework that enhances large language models (LLMs) with information retrieval capabilities. Unlike traditional AI models that rely solely on training data for inference, RAG dynamically retrieves content from internal databases, external knowledge bases, or real-time information sources, integrating the retrieved data into its responses or generated text.
This retrieval + generation hybrid approach gives RAG significant potential in areas such as enterprise knowledge management, data analysis, and automated workflows. Compared to a pure LLM, RAG ensures both accuracy and the ability to generate more precise, contextually relevant content based on actual business needs.
How RAG Works
RAG integrates two core concepts: retrieving information from external sources and generating responses using a language model. This approach enhances accuracy and clarity by leveraging external knowledge. RAG functions through a combination of retrieval and generation. When a user submits a query, a retrieval model searches an external knowledge base—such as internal company documents, databases, or web resources—to find the most relevant information. These retrieved documents are then combined with the query and passed to a pre-trained LLM. The LLM processes this enriched input, incorporating the retrieved knowledge into its response generation. This dual-step process ensures responses are contextually accurate, factually grounded, and more informative than those generated by standalone LLMs. By dynamically integrating real-time or domain-specific information, RAG reduces hallucinations and enhances the reliability of AI-driven communication and decision-making.
In a business context, RAG can efficiently search company documents, databases, or web sources to extract the most relevant information. This data is then processed by a language model to generate contextually appropriate responses. Compared to traditional search tools or standalone language models, RAG delivers more precise and insightful results, making it particularly valuable for businesses aiming to optimize information management and support data-driven decision-making.
How RAG Enhances Business Data Management
In business, decision quality depends on accurate, accessible data. RAG ensures this by fetching current information, avoiding reliance on outdated sources, and making data-driven choices more reliable. It also improves analysis, extracting insights from unstructured data like emails or reports to reveal trends and opportunities. Scalable and adaptable, RAG suits businesses of all sizes, optimizing knowledge systems for better data use.
RAG boosts efficiency by automating information retrieval, freeing staff from manual searches across systems and delivering fast, precise answers. This saves time and lets employees focus on strategic tasks. Plus, it cuts costs by handling repetitive jobs such as data sorting, summaries, compliance checks, reducing manual effort for smarter, leaner knowledge management.
How to Deploy RAG in an Enterprise?
To fully leverage RAG, businesses must begin with data organization and preprocessing, ensuring that stored information is formatted for easy retrieval and analysis. Additionally, an efficient retrieval system needs to be built, combining keyword matching and semantic understanding to ensure the accuracy and relevance of information retrieval.
The next step is integrating with a LLM. Enterprises can choose industry-specific models and fine-tune them to enhance their business relevance. It is also crucial to develop a user-friendly front-end interface to ensure RAG can be seamlessly integrated into enterprise knowledge bases, customer service systems, and data analysis platforms, making it accessible for both employees and users.
Finally, businesses should continually optimize their RAG system. As data volumes grow and business needs evolve, an effective feedback mechanism should be established. By regularly monitoring system performance, adjusting algorithms, and refining query results, businesses can ensure RAG operates efficiently in the long term.
RAG Application Scenarios in Enterprises
RAG demonstrates strong industry adaptability due to its unique modular architecture and dynamic knowledge integration capabilities, offering customized solutions tailored to the core business needs of different sectors. Its flexibility is reflected in three main areas:
- Diversity of Knowledge Sources
RAG can integrate structured data (databases, Excel), unstructured data (PDFs, emails), and real-time data (APIs, web scraping).
Examples:
- Manufacturing: Linking product design documents and supply chain databases to address quality control issues.
- Finance: Real-time retrieval of central bank policy documents and market data to generate investment recommendations.
- Scenario-Based Process Adaptation
RAG adjusts retrieval strategies (e.g., exact search/semantic search) and generation controls (e.g., precision mode/creative mode) to match industry characteristics.
Examples:
- Healthcare: Strictly referencing clinical guidelines to generate diagnostic suggestions (high precision mode).
- Marketing: Combining user profiles with trends to create creative copy
- End-to-End Scalability
Supports deep integration with existing enterprise systems (CRM, ERP).
Examples:
- Legal: Integrating with contract management systems to automatically generate risk clause analysis.
Challenges in Adopting RAG for Enterprises
While RAG offers immense potential, businesses must address the following challenges during implementation:
- Data Security and Privacy Compliance: RAG requires access to large volumes of enterprise data, so it is essential to ensure robust security measures, such as data encryption and access control, are in place to prevent data breaches or misuse.
- AI Model Bias and Hallucination: The retrieval and generation results of RAG may be influenced by the data sources, so businesses need to establish regular audit mechanisms to fine-tune the model and ensure output accuracy and fairness.
- Complexity of Technical Integration: Many enterprises still rely on traditional IT infrastructure, so integrating RAG seamlessly with existing systems becomes a key challenge. Additionally, upfront deployment costs, including computing resources, data storage, and AI processing power, must also be considered.
Conclusion:
As digital transformation accelerates, efficient data retrieval and application are crucial for competitive advantage. RAG, a next-generation AI technology, optimizes knowledge management and enhances decision-making accuracy and agility. By integrating retrieval and generation capabilities, RAG bridges the gap between data storage and intelligent application, boosting efficiency, improving decision quality, and eliminating information silos.
RAG has evolved from a concept to essential enterprise infrastructure. Leading companies are accelerating its adoption through dedicated teams, high-value scenarios, and continuous optimization. Early adopters are already seeing significant gains in efficiency and competitiveness. As RAG technology evolves, its value will only grow, making it essential for enterprises to integrate it into core business processes to stay ahead in the digital era.