What is the Model Context Protocol (MCP)?
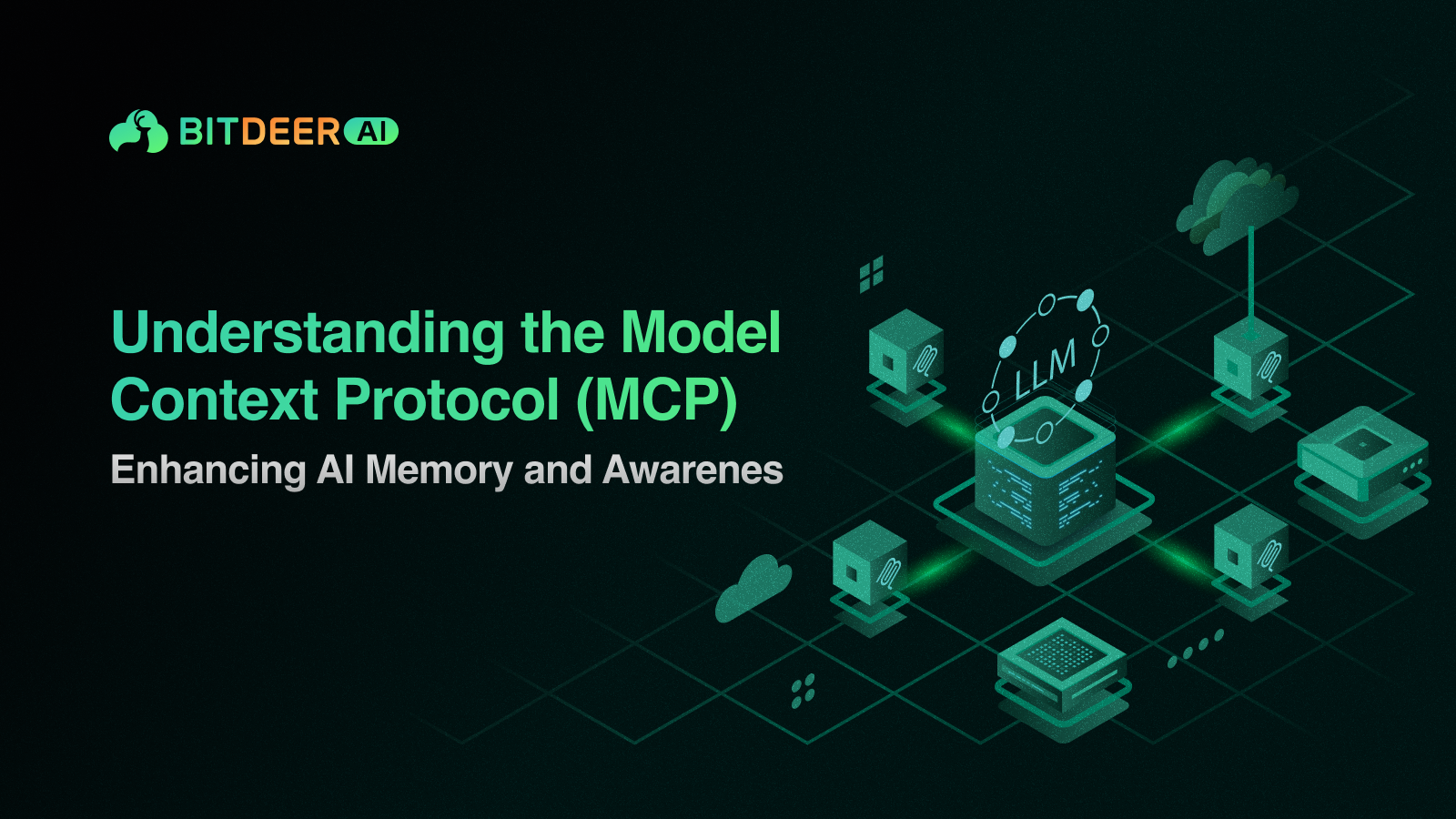
As artificial intelligence continues to evolve rapidly, the need for better coordination, interoperability, and contextual understanding among models has never been more urgent. Enter the Model Context Protocol (MCP), an emerging specification that has quickly gained traction in AI communities and tech giants alike.
By early 2025, MCP has become one of the most talked-about innovations in the open-source AI ecosystem, signaling a major shift toward standardized communication between models, tools, and agents. From open-source contributors on GitHub to major platforms like Hugging Face and OpenAI, interest in MCP is growing. The AI industry's push toward modular, composable systems and autonomous agents makes MCP not just a trend but a potential foundation for the next era of AI development.
The protocol was originally introduced by Anthropic in November 2024. Anthropic unveiled MCP as an open-source initiative aimed at enabling consistent, transparent, and modular integration between AI models and external tools or data sources.
Why Do We Need MCP?
MCP reflects Anthropic’s broader vision of building transparent, modular AI systems that can handle complex, multi-step reasoning and sustain long-term interactions reliably. This vision addresses a growing challenge in the AI ecosystem: as models, APIs, tools, and user interfaces increasingly interact in complex workflows, the lack of shared context can lead to inefficiencies and inconsistencies.
In a world where multiple components must work together seamlessly, context fragmentation becomes a critical issue. Systems often operate in isolation, lacking a shared memory or understanding of what happened previously. Some of the major challenges MCP aims to solve include:
- Lack of Interoperability: Different models, services, and tools often fail to communicate effectively.
- Context Confusion: There is no universal method to track what information has already been exchanged.
- Agent Collaboration Issues: Multi-agent setups need a structured way to share progress, roles, and responsibilities.
- Tool Integration Complexity: LLMs using plugins, APIs, and external services need consistent Input/Output and execution context.
To better illustrate this, consider the diagram below: without MCP, integrating different LLMs and products requires complex and often cumbersome relationship management.
What is MCP and What Are Its Key Elements?
MCP is a standardized framework that defines how context is represented, shared, and updated across different components in an AI system. A protocol is essentially a set of rules for formatting and processing data. As the name suggests, MCP is indeed a protocol, specifically, a set of rules that standardize how large language models (LLMs) connect to external sources of information. It acts as the glue that allows various elements such as language models, tools, agents, and memory stores to interoperate smoothly.
MCP adopts a client-server architecture and uses the JSON-RPC 2.0 protocol to facilitate communication between model applications and external data services. Its architecture comprises three primary components:
- MCP Host: This refers to the AI-powered application that initiates connections and sends queries to MCP servers. Examples include chat clients or custom-built AI agents that need to access external data or functionality.
- MCP Client: Running inside the host application, the MCP Client manages communication with one or more MCP servers. It handles connection setup, sends requests, and receives responses. A single AI application (host) can connect to multiple MCP clients simultaneously to access different capabilities provided by various servers.
- MCP Server: This component offers specific functions or data by exposing services to MCP Clients through the standardized protocol. It interacts with local data sources or remote APIs to fulfill requests sent by clients.
In simple terms, the Host initiates the task, the Client handles communication, and the Server delivers the required capabilities. These components work together to enable AI applications to access and utilize external resources efficiently, promoting interoperability and reducing the need for custom integrations. MCP ensures that whether you're building a customer service chatbot, a multi-agent research assistant, or an AI productivity suite, all components are on the same page.
How MCP Works: A Conceptual Example
Let’s consider a customer support agent built with Claude that can access a knowledge base, issue refund tickets, and escalate to a human if necessary.
- Initialization:
- A user query triggers an MCP context with identity (user ID), channel (web chat), and intent (support).
- Goal Structuring:
- MCP encodes the top-level goal: "Resolve user complaint."
- Sub-goals might include: "Retrieve product history," "File refund," or "Send confirmation."
- Tool Invocation:
- Claude interacts with a ticketing API. MCP records the request, status, and results.
- Memory Update:
- As the case progresses, MCP continuously updates context to reflect completed tasks and user sentiment.
The process begins with a user query submitted through the Claude Desktop (Host), which is routed to the Claude model. If file access is required, the MCP Client initiates a connection to the appropriate File System MCP Server, executes the requested operation, and returns the result. The Claude model then generates a response, which is presented within the Claude Desktop interface.
This structured process ensures transparency, traceability, and modularity across all AI components. Moreover, this architectural design enables Claude to flexibly invoke various tools and data sources across different scenarios, while developers only need to focus on building the corresponding MCP Servers without needing to worry about the implementation details of the Host or Client.
MCP’s Impact on AI and Agent-Based Development
The adoption of MCP has far-reaching implications for how AI systems are built, maintained, and scaled.
Key Benefits:
- Enables Autonomous Agents: MCP makes it feasible for autonomous agents to share knowledge, collaborate, and adapt their roles dynamically.
- Simplifies Developer Workflows: Developers no longer have to manually engineer state-passing logic.
- Supports Multi-Modal Fusion: Language, vision, audio, and tabular data models can now share context more easily.
- Boosts Safety and Alignment: Clear context history improves interpretability and reduces unexpected behavior.
Final Thoughts
As AI systems become more embedded in real-world workflows, the need for shared memory and coordination continues to grow. MCP provides a unified standard, like a USB-C for AI, that allows models to connect with external tools and data sources in a consistent, open, and flexible way. It benefits users by offering powerful tools without technical complexity, and empowers developers with a clear SDK and architecture for building integrations.
Though still emerging, MCP is already being adopted by AI leaders for research agents, copilots, and assistants such as Microsoft and AWS. Its extensible design is turning today’s fragmented tools into tomorrow’s seamless AI ecosystems. A unified standard like MCP also creates a strong foundation for faster, more collaborative progress across the industry.
If your work involves multi-turn tasks, tool integration, or agent collaboration, MCP is worth exploring. The future of AI depends on smarter coordination and MCP is leading the way.